Elasticsearch+Kibanaで参議院選挙を振り返る #elasticsearch
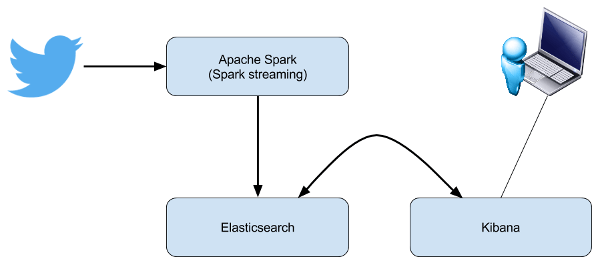
この記事は1年以上前に投稿されました。情報が古い可能性がありますので、ご注意ください。
こんにちは。木内です。先日の参議院選挙では自民党が改選議席の過半数を獲得し、勝利しました。昨今ではネットを活用した選挙活動も活発に行われています。
そこで今回はElasticsearchを活用して参議院選挙を振り返ってみましょう。
はじめに
まずは分析のデータソースが必要です。今回はTwitter社が提供しているPublic stream( https://dev.twitter.com/streaming/public )を使用しました。Public streamの受信とElasticsearchへの書き込みにはApache Sparkを使用しています。以下は簡単なシステム構成図です。
Elasticsearchに取り込んだツイートはKibanaで簡単に見ることができます。以下はKibanaでPublicストリームのツイートを表示した例です。
Twitterで「選挙」が含まれるツイートを検索する
では保存したツイートを活用していろいろな情報を探してみましょう。まずは簡単に"選挙"が含まれるツイートを探してみます。Kibanaの検索欄に以下のようにタイプすると、ツイート本文に"選挙"が含まれるツイートの一覧を抽出することができます。
messages: "選挙"
以下が検索結果です。・・・なんか妙に少ないですね。
Kibana画面右上の検索範囲を調整することで検索範囲を広げることができます。デフォルトでは「last 15 minutes」になっているので、「last 60 days」まで広げて再度検索してみます。
だいぶヒットするようになりました。Kibana画面上には日時ごとにどの程度のヒットがあるかを視覚的に表示しています。検索にヒットした件数(ツイート数)は約140,000件です。見ると、6月18日と、7月10日に多くのツイートが行われているようです。7月10日は投票日でしたのでツイートが多いのは合点がいきますが、6月18日には何があったのでしょうか。棒グラフをクリックすると、その日時のデータをさらに抽出します。
選挙は選挙でも「AKB48総選挙」がヒットしていました。これは今回の趣旨とは異なりますのでフィルタしましょう。以下のような検索条件で、再度ツイートを抽出してみます。
message: "選挙" AND -message: AKB48 AND -message: "総選挙"
だいぶ意図通りの結果が出るようになりました。改めて過去60日で選挙に関するツイートを抽出してみます。
ヒット数は全体で約100,000ツイートでした。これはPublic streamなのでツイートのすべてが取得できているわけではありませんが、Twitter全体の中での傾向もおおよそ似たようなものになっているかと思います。
また投票日当日のツイート数が最も多く、次いで6月22日ごろからじわじわとツイート数が増えています。6月22日は公示日でしたので、選挙戦の始まりとともにツイートが増えていることがわかります。投票日翌日のツイートも多いですね。これは選挙の結果についてツイートしているのでしょう。その後3日ほどで選挙に関するツイート数は6月22日以前の状況に戻っています。今回の選挙は投票率が低く、あまり関心は高くなかったそうなので、みなさんの関心はすぐに他に移ってしまったのかもしれません。
当選議員数とツイート数の関係
つづけて各政党についてツイートされた数と、実際の結果を比較してみましょう。読売新聞のページ(http://www.yomiuri.co.jp/election/sangiin/2016/?from=ycnav3)によると、今回の参議院選挙における当選数は以下のようになっています。
政党名 | 当選議員数(今回当選を含めた合計議席数) | 割合(合計議席数の割合) |
自由民主党 | 55(120) | 45.5%(49.6%) |
民進党 | 32(49) | 26.4%(20.2%) |
公明党 | 14(25) | 11.6%(10.3%) |
共産党 | 6(14) | 5.0%(5.8%) |
おおさか維新の会 | 7(12) | 5.8%(5.0%) |
社会民主党 | 1(2) | 0.8%(0.8%) |
生活の党と山本太郎となかまたち | 1(2) | 0.8%(0.8%) |
無所属/その他 | 5(18) | 4.1%(7.4%) |
合計 | 121(242) |
さて、では上記で抽出した選挙に関するツイート(合計数: 101,889件)にさらに政党名でフィルタをかけてみましょう。以下のようなクエリを実行します。
(message: "選挙" AND -message: AKB48 AND -message: "総選挙") AND (message: "自民党" OR message: "自由民主党" OR message: "自民")
以下は各政党でフィルタした際のツイート数の一覧です。割合の母数は、政党名に言及しているツイート数の合計(25,345件)です。
政党名(他にキーワードとして使用したもの) | ツイート数 | 割合 |
自由民主党(自民党, 自民) | 8695 | 34.3% |
民進党(民進) | 4722 | 18.6% |
公明党(公明) | 2225 | 8.8% |
日本共産党(共産) | 5075 | 20.0% |
おおさか維新の会(維新の会, 維新) | 1704 | 6.7% |
社会民主党(社民党, 社民) | 1429 | 5.6% |
生活の党と山本太郎となかまたち(生活の党, 生活) | 1495 | 5.9% |
グラフにしてみると以下のような感じになっています。
驚くべきことに、政党の当選議員数と政党名に言及したツイートの割合には相関が見られます。細かく見ると以下のようなことがわかります。
- 共産党についてはツイート数と当選議員数との間に相関がないように思われる
- 社民党、生活の党、おおさか維新の会についてはおおよそ同率のツイートがあったが、当選議員数には違いが出た。
さらに深く分析をすすめるには
今回の例はTwitterのようなSNSでの意見表明が現実の世界にどのように関連しているかを示す一つの例です。より深く分析をすすめるとしたら、以下のようなことに配慮してみるとよりよい結果を導くことができるかもしれません。
- ツイートの対象(今回の場合は政党)に対する感情分析を交えるとより分析の精度を上げることができるかもしれません。政党に言及したツイートであっても、実際にはその政党を批判したり、こき下ろしたりする内容であることもあるためです
- 昨今では議員個人がTwitterアカウントを取得して情報発信をしています。どの議員に言及しているか。言及している人がどの政党や議員をフォローしているかを分析に加味すると、より興味深い結果を導くことができるかもしれません
さいごに
ElasticsearchとKibanaを組み合わせることで、強力なデータベースと、自然言語による検索を行うことができます。何かを分析する場合にとても強力なツールになるでしょう。またApache Sparkを使用することでTwitterのようなストリームデータを継続的にElasticsearchに保存することができます。
今回の例は非常に簡単なものですが、より活用をすすめることで面白い結果を出すことができるかもしれません。もしお気づきのことがありましたらお知らせいただければ幸いです。読んでいただくなかで何か新しい発見があったのであればとても嬉しいです。